Danh sách các nhà cái tặng tiền miễn phí khi đăng ký 2024 cho tân thủ khi khởi tạo tài khoản mới, chia sẻ các code trải nghiệm tại nhà cái uy tín, hướng dẫn nhận khuyến mãi freebet, các nhà cái tặng tiền không cần nạp, các nhà cái khuyến mãi trải nghiệm, tặng 38k, 50k, 66k, 88k, 100k, 128k, 150k, 200k chơi slot nổ hũ, casino, bắn cá, cá độ bóng đá..
Top Nhà Cái Tặng Tiền Cược Miễn Phí 2024 (01/05/2024)
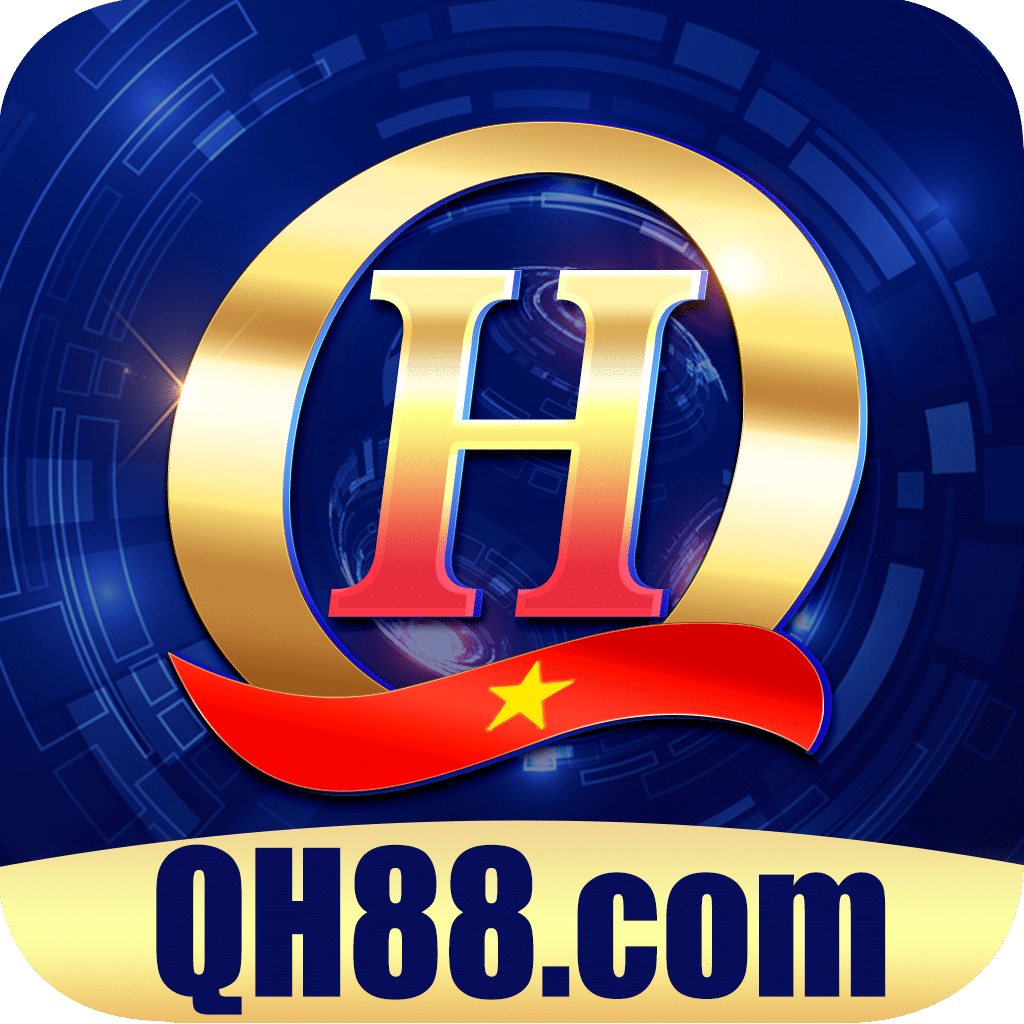
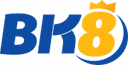
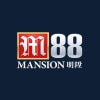

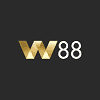
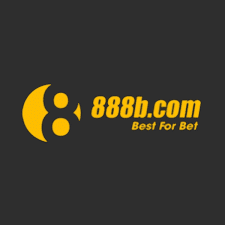
Tin nhà cái mới nhất 01/05/2024
Top 15 Nhà Cái Uy Tín Nhất Việt Nam 2024 (Cập Nhật Hôm Nay)
+23 Nhà Cái Lừa Đảo 2024 – Cách Nhận Biết Để Tránh Xa
+99 Nhà Cái Mới Nhất (Mới Ra Mắt) 2024
⚔ Cần chú ý ⚔ !!!
❌❌❌ Để tránh bị lạm dụng khuyến mãi, số lượng code miễn phí hoặc tiền trải nghiệm free sẽ được giới hạn số lượng và chỉ phát cho các khách hàng tiềm năng – nạp tiền, chơi thật, cược thật. Việc tạo nhiều tài khoản ảo để chơi 100% sẽ bị khóa và chỉ khiến bạn mất thời gian.
🎁🎁🎁 Sau khi đăng ký, vui lòng chat với CSKH của nhà cái để được lên điểm khuyến mãi. Cập nhật khuyến mãi nhà cái hàng ngày tại trang chia sẻ code nhà cái bonesuk.com
✅✅✅ Nên chơi tại các nhà cái lớn, uy tín nhiều năm, thưởng chào mừng và khuyến mãi đều, chung chi sòng phẳng, xanh chín.
🎲🎲🎲♢♧♡♤ 🎲🎲🎲
Tiền cược miễn phí là chiêu bài của nhà cái để thu hút người chơi mới. Hình thức khuyến mãi này rất phù hợp cho các anh em muốn trải nghiệm game hoặc muốn “khởi nghiệp” với số vốn 0 đồng. Tuy nhiên, không dễ để có thể nhận được cược miễn phí. Thông thường top nhà cái tặng tiền cược free sẽ yêu cầu nhiều điện kiện kèm theo, và cũng không ít các nhà cái “treo đầu dê bán thịt chó”, quảng cáo 1 đằng nhưng đăng ký xong thì không có tiền cược, hoặc cược thắng thì không cho rút.
Thị trường cá cược trực tuyến tại Việt Nam hiện tại đã khá bão hòa với hàng chục, hàng trăm trang web cá cược mới ra mắt, quảng cáo rầm rộ và thương hiệu nào cũng tự cho mình là “uy tín” và “số một”. Trong đó không ít nhà cái non trẻ có dấu hiệu lừa đảo, xù tiền người chơi. Do đó các cược thủ cần kiểm tra kĩ lưỡng trước khi nạp tiền vào chơi. Dưới đây là top 15 nhà cái tặng tiền cược miễn phí mới nhất 2024 và các thông tin quan trọng mà bạn cần biết khi nhận thưởng miễn phí.
Lưu ý: các khuyến mãi dưới đây chia thành 2 loại:
- Khuyến mãi chung: tiền cược miễn phí khi đăng ký mới tài khoản dành cho tất cả các thành viên (nhà cái tặng tiền trải nghiệm 2024 – nhà cái thưởng tiền chơi thử).
- Khuyến mãi riêng của đại lý: đăng ký dưới mã đại lý được freebet riêng do đại lý đó cấp.
Mục Lục
Top 15 nhà cái uy tín tặng tiền miễn phí khi đăng ký 2024
Dưới đây tôi sẽ điểm mặt top 15 nhà cái tặng thưởng uy tín đã qua kiểm chứng.
1. QH88 tặng 188k, 100% khi đăng ký thành viên mới
QH88 là thương hiệu cá cược online hợp pháp được cấp phép bởi PAGCOR, thuộc quản lý của tập đoàn Ekxinum, Inc. Nhà cái QH88 có tiềm lực tài chính mạnh mẽ, độ uy tín cao, hoạt động đa quốc gia và thu hút hàng triệu bet thủ tham gia đặt cược hàng tháng.
QH88 là nhà cái mới ra mắt đang “gây bão” trong cộng đồng cá cược trực tuyến tại Việt Nam. Nhà cái này có rất nhiều chính sách khuyến mãi hấp dẫn, phù hợp với anh em tân thủ hoặc các tay chơi muốn đổi nhà cái để tìm vận may mới.
QH88 hiện có đang có rất nhiều khuyến mãi:
- KHUYẾN MÃI 100% NẠP ĐẦU
- BẢO HIỂM VỐN ĐẾN 20 TRIỆU ĐỒNG
- THƯỞNG THÊM 5% NẠP MỖI CHỦ NHẬT
- THƯỞNG THÊM 100% TIỀN NẠP CHO THÀNH VIÊN CŨ TẠI PHIÊN BẢN CAO CẤP MỚI
- HOÀN TRẢ KHÔNG GIỚI HẠN
LINK NHẬN TRẢI NGHIỆM QH88 : tại đây
Lưu ý: Nhà cái chỉ tặng code hoặc hoàn trả cho các tài khoản tiềm năng, nghĩa là tài khoản thật, người chơi thật. Các tài khoản trục lợi hoặc tài khoản ảo thường sẽ không được nhận. Với các tài khoản chơi thường xuyên, đại lý sẽ hoàn trực tiếp tiền vào tài khoản đó.
2. SHBET tặng 888k, siêu khuyến mãi, mưa quà tặng
SHBET là nhà cái mới nổi 2024, đang có sức phát triển hết sức mạnh mẽ. SHBET được biết đến là thương hiệu liên quan tới những ông lớn trong ngành như 789BET/ AE888/ KUTHABET. Cách vận hành của game cũng khá giống với những trang này. Do đó bạn hoàn toàn có thể yên tâm về độ tin cậy, tiềm lực tài chính cũng như độ minh bạch của trang.
Shbet đang có hàng loạt khuyến mãi cực khủng nhằm thu hút người chơi. Thông thường, những nhà cái mới sẽ không tiếc tiền khuyến mãi, quảng cáo để lôi kéo người chơi mới. Do đó, bạn có thể tận dụng để có 1 khởi đầu dễ dàng hơn với các khuyến mãi tân thủ, khuyến mãi tiền nạp.
Link nhận 888k khuyến mãi SHBET tại đây
Shbet cũng có nhiều hình thức khuyến mãi để bạn tận dụng tăng số dư tài khoản, như giới thiệu thành viên để nhận quà. Các event cũng diễn ra liên tục, bạn có thể có cơ hội nhận tiền, phần quà hiện vật như xe SH, iphone… Mỗi tuần, SHBET cập nhật hàng chục khuyến mãi mới. Nếu bạn là “Fan” của việc “bào khuyến mãi” nhà cái thì chắc chắc SHBET là lựa chọn lí tưởng nhất ở thời điểm này.
Shbet không có khuyến mãi tặng cược miễn phí khi đăng ký cho thành viên mới mà chỉ có khuyến mãi tiền nạp lên tới 888k. Tuy nhiên, Betway phát triển theo hướng đại lý nên nhiều đại lý sẵn sàng tặng code 28k, 38k, 58k, 100k, 128k cho bạn trải nghiệm. Việc của bạn là chỉ cần đăng ký tài khoản qua mã đại lý của họ.
3. 123B – nhà cái tặng tiền không cần nạp
123B là một nhà cái lớn hàng đầu ở Châu Á và có uy tín từ rất lâu đời. Nay 123B đang phát triển rất mạnh mẽ ở thị trường Châu Á, nhất là thị trường Việt Nam. 123B mạnh về sản phẩm casino và lô đề xổ số với tỉ lệ thưởng cực cao, hoàn trả lớn và nhiều ưu đãi khủng dành cho thành viên mới..
Về độ uy tín thì 123B nhận số 2 chắc ít ai dám nhận số 1. 123B có tiềm lực siêu mạnh, thường xuyên triển khai nhiều sự kiện offline hấp dẫn, các chương trình thiện nghiện, hoạt động xã hội cho sinh viên, người nghèo… 123B rất mạnh ở thị trường châu Á, đặc biệt là sản phẩm cá độ bóng đá, sòng bạc trực tuyến, slot game, bắn cá.
Hiện tại 123B có chương trình tặng tiền cược miễn phí cho hội viên mới trải nghiệm, chỉ cần đăng ký, xác minh tài khoản, thông tin cá nhân là có ngay điểm cược free trong tài khoản.
LINK NHẬN TRẢI NGHIỆM 123B :→ 🎁 NHẬN CODE TẠI ĐÂY
Để có thể nhận ngay 90.000 VNĐ từ 123B thì anh em cũng cần phải điền đầy đủ thông tin và xác nhận chúng như tôi đã nói ở trên. Ngoài ra còn một số điều kiện riêng của 123B như là cập nhật vào tài khoản chính với số tiền là 200.000 VND, rút tiền thành công 300.000 VND và tiền thưởng cần phải trải qua 1 vòng cược trước khi anh em thực hiện giao dịch rút tiền cược miễn phí về tài khoản mình nha. Cũng không quá khó để nhận 90.000 từ đúng không nào?
4. AZ888 – Đăng ký tặng tiền trải nghiệm + thưởng nạp đầu cực cao
AZ888 hay nhà cái số đỏ đang là cái tên gây được nhiều ấn tượng nhất trong làng giải trí đổi thưởng online năm 2024, 2024. AZ888 đầu tư rất mạnh cho quảng cáo, truyền thông, marketing, bạn có thể thấy độ phủ thương hiệu cực lớn của Sodo trên Internet.
AZ888 có thế mạnh về sản phẩm lô đề xổ số với tỉ lệ ăn cực cạnh tranh – 1 ăn 99.52. Ngoài ra AZ888 cũng có nhiều sản phẩm cá cược hấp dẫn khác như thể thao (8 sảnh cược), Casino (12 sảnh cược), Slot, Bắn Cá, Game bài. Tất cả đều được thiết kế đẹp mắt, game chơi cực mượt và sinh động, ít phát sinh lỗi hay giật lag.
Link nhận khuyến mãi từ AZ888 tại đây:
Hiện tại AZ888 Casino có nhiều khuyến mãi hấp dẫn cho anh em trải nghiệm như:
- Đăng ký nhận ngay 18k tới 188k miễn phí
- Nhận lì xì miễn phí lên tới 888.888.888 VNĐ
- Vòng quay may mắn nhận quà giá trị
- Điểm danh mỗi ngày, nhận ngay tiền thưởng
- Giới thiệu bạn bè nhận 88k
- Hoàn trả lên tới 3%
Link nhận khuyến mãi từ AZ888 tại đây
5. BK8 khuyến mãi 200% nạp đầu
BK8 là cái tên hot nhất trên thị trường cá cược trực tuyến 2024 với sự đầu tư mạnh mẽ về mặt truyền thông, marketing. BK8 hiện là nhà tài trợ cho CLB NORDWICH CITY và có John Terry, Robin Van Persie làm đại sứ thương hiệu, chứng tỏ tiềm lực rất mạnh và xứng đáng cho anh em chọn mặt gửi vàng.
BK8 có trụ sở tại đảo quốc Malta, hoạt động ở trên 10 thị trường và được đánh giá rất cao bởi hầu hết các tổ chức minh bạc cờ bạc quốc tế.
Ngoài ra BK8 còn có nhiều khuyến mãi khác như thưởng chào mừng lên tới 200%, thưởng nạp lại hàng ngày lên tới 20%.
6. EE88 tặng 100%, hoàn trả không giới hạn
EE88 cũng là một nhà cái có tiếng được nhiều anh em bạc thủ biết đến. EE88 nổi tiếng nhờ chất lượng và dịch vụ tận tình, chu đáo, kịp thời, luôn mang đến cho người chơi những gì tốt nhất. Bên cạnh đó EE88 còn tự hào là một trong những nhà cái trang bị nhiều phương thức thanh toán giao dịch nhất cho người chơi để khách hàng luôn có những trải nghiệm tốt nhất.
EE88 hiện có đang có rất nhiều khuyến mãi:
- KHUYẾN MÃI 100% NẠP ĐẦU VÀ 50% NẠP LẦN 2 TỐI ĐA 1TR888
- BẢO HIỂM VỐN ĐẾN 20 TRIỆU ĐỒNG
- THƯỞNG THÊM 5% NẠP MỖI CHỦ NHẬT
- THƯỞNG THÊM 100% TIỀN NẠP CHO THÀNH VIÊN CŨ TẠI PHIÊN BẢN CAO CẤP MỚI
- HOÀN TRẢ KHÔNG GIỚI HẠN
LINK NHẬN TRẢI NGHIỆM EE88 : tại đây
7. JUN88 – tặng 88,88,000, giới thiệu bạn bè được thưởng tiền chơi thử
JUN88 là trang cá cược bóng đá nổi tiếng nhất Vương quốc Anh. Isle of Man Gambling Supervision Commission đã cấp giấy phép hoạt động và chứng nhận quốc tế cho JUN88. Nhà cái này đã không ngừng vươn lên trở thành thương hiệu hàng đầu trong ngành cá cược trực tuyến.
ĐĂNG KÝ JUN88 NHẬN THƯỞNG TRẢI NGHIỆM: → 🎁 Nhận code tại đây
Điều kiện nhận KM trải nghiệm chơi thử tại JUN88:
- Đăng ký, xác minh tài khoản chính chủ, liên kết ngân hàng
- Mã Khuyến Mãi: FREEVIP
8. P3 tặng 8888k, tặng ngẫu nhiên 58k cho thành viên mới
Cùng lên P3 làm vài trận game cho máu nè anh em ơi. Nói gì chứ về P3 thì khỏi bàn rồi trang web game online thuộc những trang web sở hữu người chơi khá là đông đảo bởi vì trên đây có rất là nhiều game hay cho các bạn lựa chọn.
Nhận khuyến mãi P3 ngay: → 🎁 LINK TẠI ĐÂY
P3 là Nhà cái Quốc Tế được điều hành bởi Tập Đoàn Premier Gateway International được cấp giấy phép hoạt động cờ bạc trực tuyến hợp pháp bởi PAGCOR. Tập đoàn giải trí này sở hữu nhiều thương hiệu Nhà cái nổi tiếng ở khắp châu Á. P3 chính thức có mặt tại thị trường Việt Nam từ năm 2020.
P3 cung cấp đa dạng các trò chơi từ live Casino, Đá gà, Bắn cá, Slot, Thể thao, game bài. Ưu điểm của trang là nạp rút nhanh, hỗ trợ đa nền tảng, app rất nhẹ và mượt, ít lỗi, giao diện đẹp mắt. Chất lượng chăm sóc khách hàng cũng rất tốt, chuyên nghiệp và hỗ trợ 24/7.
NHỮNG ƯU ĐÃI NỔI BẬT
Các chương trình khuyến mãi thường xuyên được cập nhật, tạo sức hút lớn đối với cộng đồng người chơi. Đặc biệt từng khu vực trò chơi, người chơi đều có những ưu đãi tương ứng.
Dù bạn là người chơi mới hay tham gia chơi lâu năm thì đều được hưởng những chính sách ưu đãi hấp dẫn. Điều này tạo nên một sân chơi công bằng, hiệu quả.
Hãy đến với P3 ngay chứ chần chừ gì anh em ơi !!! “Chơi vui đem tiền về nhà liền tay”.
Link đăng ký nhận 58k miễn phí tại P3 tại đây: → 🎁 LINK TẠI ĐÂY
9. 8xbet – tặng 100k khi đăng ký
8xbet (tiền thân là 978bet) là một lính mới trong làng cá cược, gia nhập vào đầu năm 2024. Nhà cái này đã thu hút được sự quan tâm của rất nhiều game thủ ngay từ khi mới ra mắt. 8XBET là một nhà cái cá cược trực tuyến có trụ sở tại Philippines được cấp phép bởi ba tổ chức trò chơi được công nhận: Malta MGA, PAGCOR và GC (Gambling Commission). Sở hữu lý lịch và điều kiện tài chính hùng mạnh, KOK thực sự đã thuyết phục được những bạc thủ khó tính nhất.
LINK NHẬN 100K 8XBET TẠI ĐÂY
Với 8XBET thì để nhận 100.000 VND, bạn cần hoàn thành đầy đủ thông tin xác minh danh tính, số điện thoại, Email, thông tin thẻ ngân hàng là đã có thể nhận được 100.000 VND này mà không yêu cầu tổng cược. Vì thế anh em có thể rút tiền ngay sau khi hoàn thành 10 vòng cược và có số dư tài khoản ít nhất 1000k.
10. 68gamebai tặng 100k khi đăng nhập tài khoản 3 ngày
68GAMEBAI được thành lập vào năm 2021 và chuyên hoạt động kinh doanh nhiều loại trò chơi khác nhau. 68GAMEBAI ủy quyền giám sát tất cả các sản phẩm và dịch vụ cho First Cagayan Leisure and Resort Corporation (www.firstcagayan.com) tại Đặc khu Kinh tế Sông Cagayan của đất nước Philippines, đất nước đáng tin cậy được nhiều nhà cái lựa chọn làm trụ sở.
Khuyến mãi hot từ 68GAMEBAI – đăng nhập thường xuyên nhận quà liên tục
- Ký tên đủ 3 ngày, nhận ngay 100,000.00 VND
- Ký tên đủ 7 ngày, nhận ngay 300,000.00 VND
Link nhận khuyến mãi 100k 68GAMEBAI tại đây
Vẫn là những yêu cầu chung quen thuộc anh em cần phải làm là xác minh thông tin đầy đủ, chính xác. Ngoài ra với 68GAMEBAI thì nhà cái này còn yêu cầu anh em phải tham gia 10 vòng cược để nhận 100k tiền cược miễn phí. Và để rút tiền thì cần phải nạp số tiền bằng đúng số dư tài khoản, tham gia thêm 1 vòng cược nữa trong vòng 7 ngày thì mới có thể hoàn toàn có được 100k.
11. ME88 tặng 100k trải nghiệm miễn phí
ME88 là nhà cái có mặt lâu đời ở nhiều quốc gia nhưng mới ra mắt ở thị trường Việt Nam từ 2022. ME88 là tên tuổi khủng với tiềm lực tài chính hết sức mạnh mẽ. Hiện tại, ME88 có đại sứ thương hiệu là MC Gregor – tay đấm UFC nổi tiếng #1 hiện nay.
Link nhận khuyến mãi ME88 tại đây
Me88 là game tặng tiền miễn phí đáng để trải nghiệm nhất hiện nay, mức thưởng 100k trải nghiệm này áp dụng cho hội viên nạp 200k vào tài khoản đầu tiên.
Me88 có đa dạng sản phẩm cá cược cho bạn lựa chọn, với mức độ đầu tư của ME88 vào thương hiệu, hình ảnh và khuyến mãi thì bạn hoàn toàn có thể yên tâm lựa chọn trang web tặng tiền không cần nạp này để chơi thử.
12. HB88 – nhà cái thưởng tiền chơi thử
Dù chỉ mới ra đời cách đây vài năm nhưng HB88 đã nhanh chóng khẳng định mình là một trong những nhà cái nổi bật nhất Châu Á, thu hút một lượng lớn người chơi. HB88 có chứng chỉ pháp lý từ Costa Rica và PAGCOR của chính phủ Philippines cung cấp sự đảm bảo về tính công bằng. HB88 là nhà cái cá cược lớn nhất trong ngành công nghiệp cá cược hiện nay, cung cấp một số khoản tiền thưởng hấp dẫn và một loạt các sản phẩm cá cược.
Khuyến mãi hot từ HB88:
- Đăng ký tặng 100k + 58tr
- Thưởng chia sẻ 88 – 888k
- Điểm danh mỗi ngày nhận thưởng ngẫu nhiên 0.01 – 30 điểm
- Bảo hiểm vốn với đơn cược đầu tiên
Link nhận 100K miễn phí tại HB88
Người chơi HB88 sẽ được tận hưởng tất cả các dịch vụ tốt nhất của nhà cái, nhờ vào đội ngũ nhân sự có năng lực, nổi tiếng và sáng tạo đã phát hành một loạt các trò chơi phổ biến.
Để nâng cao chất lượng dịch vụ và mang đến cho người chơi những trải nghiệm game tốt nhất. Sau khi đã xác thực tài khoản với đầy đủ thông tin yêu cầu xong là anh em đã có thể nhận ngay phần thưởng là 100 điểm tương ứng với 100.000 VND. Số lượng code sẽ giới hạn mỗi ngày nên anh em nhanh tay để nhận tiền cược miễn phí nhé.
13. FCB8 thưởng 50k
FCB8 được thành lập năm 2019 với sự cấp phép của Electraworks Ltd và được bảo hộ bởi hội đồng cờ bạc Gibraltar Gambling Commission – GGC. Đây là nhà cái cá cược nổi tiếng nhất châu u trong lĩnh vực giải trí cá cược trực tuyến, cung cấp nhiều loại trò chơi cá cược, bao gồm thể thao, thể thao điện tử, sòng bạc trực tuyến, xổ số và trò chơi xèng,… với tỷ lệ thắng lớn nhất trên thị trường hiện nay. Thực hiện mục tiêu mang đến một sân chơi cá cược đẳng cấp quốc tế nổi bật cho thị trường Châu Á nói chung và Việt Nam nói riêng.
Điều kiện để anh em nhận 50.000 VND tiền cược miễn phí đó là đăng ký xác minh đầy đủ thông tin cá nhân của mình. Sau đó anh em phải nạp thêm 100.000 VND và tham gia 1 vòng cược 150.000 VND thì mới có thể rút tiền.
14. TF88 tặng 50k
TF88 là một nhà cái cá cược trực tuyến đã được cấp giấy phép cá cược ở nước ngoài bởi cơ quan Philippines North Cagayan (NCGAC). TF88 có một loạt các trò chơi cá cược trực tuyến, bao gồm sòng bạc trực tuyến, cá cược thể thao, poker, game slots, xổ số, esports và bắn cá. Các trò chơi tại TF88 được cung cấp bởi các nhà cung cấp được công nhận trên thị trường cá cược.
Để có thể nhận tiền thưởng miễn phí thì anh em cần đăng ký, xác minh đầy đủ thông tin và có tổng giao dịch gửi tiền tối thiểu 320.000 VND trong ngày bằng thẻ cào điện thoại. Và để rút tiền này thì anh em phải trải qua 6 vòng cược mới được rút nhé.
15. Aw8 – top nhà cái tặng tiền cược free
Aw8 hay AceWin8 là công ty cá cược trực tuyến lớn nhất Châu Á, cung cấp cho khách hàng các thương hiệu thể thao, sòng bạc, xổ số và trò chơi nổi tiếng nhất Châu Á. Aw8 là đại lý chính thức cho các thương hiệu cờ bạc lớn của Châu Á như CMD368, Allbet, Playtech, Spadegaming và những thương hiệu khác.
Anh em vẫn cần chú ý điền đầy đủ và thật chính xác những thông tin cá nhân để có thể nhận được khuyến mãi trải nghiệm từ Aw8 nhé!
Nhà cái thưởng đăng ký thành viên mới 50k
- VN88: đại lý VN88 tặng 50k, nhà cái VN88 tặng 50k khi gửi tiền qua di động. → vn88.vin
- BK8 tặng 50k chơi thử → 🎁 nhận thưởng tại đây
- FB88: tặng thẻ cào → 🎁
FB88.com - VWin: tặng 50k + 200% tiền nạp → 🎁
https://vietvwin.com - Bwing: tặng 50k + 100% tiền nạp đầu → 🎁
https://thethao50k.com
Top nhà cái tặng 66k – 68k
- Vwin:→🎁 code riêng của đại lý
- BK8:→🎁 Nhận code tại đây
Nhà cái khuyến mãi thành viên mới 88k
- VN88:→ 🎁vn88.vin
- T8BET:→ 🎁
t8bet.com - TF88
Top nhà cái tặng 100k khi đăng ký
Các nhà cái tặng 100k khi đăng ký mới nhất, chỉ cần tải app hoặc đăng ký tài khoản là có thể nhận tiền chơi thử:
- V9bet :→ 🎁 Nhận code tại đây
- 978bet :→ 🎁 Nhận code tại đây
- Jun88: → 🎁 Nhận code tại đây
- SV388 → 🎁 Nhận code tại đây
- 33BET:→ 🎁 Nhận code tại đây
- i9bet.com :→ 🎁 Nhận code tại đây
Top nhà cái tặng 200k miễn phí 2024
- K9win:→ 🎁 k9vn.com
- BK8:→ 🎁
bk8c.com
Thưởng 188k khi giới thiệu bạn bè:
Game slot tặng tiền cược miễn phí 2024
Hầu hết các nhà cái tặng cược miễn phí đều dành cho 2 sản phẩm chính là slot và casino, vì với điều kiện vòng cược thì tỉ lệ thắng khá thấp. Tuy nhiên, có các trò quay slot siêu rẻ như slot 888 fortunes, ăn khế trả vàng, rồng vàng… có thể tận dụng tốt điều này. Nếu may mắn, bạn hoàn toàn có thể chiến thắng và rút tiền thưởng.
Đối với game slot tặng tiền cược miễn phí, bạn có thể săn tiền cược freebet hoặc vòng cược miễn phí của nhà cái.
Top nhà cái tặng vòng quay miễn phí:
- Oppa888:→ 🎁 vi.oppa888.com/freespin-15-times
- HL8:→ 🎁 hl8daily.com
- 12bet:→ 🎁win8118.com
- Empire777 :→ 🎁 empire777.com
- 22bet :→ 🎁 22bet.com
- M8win:→ 🎁m8winvip.com
Top game bài tặng code cho tân thủ chơi slot miễn phí:
- CF68
- Manclub
- Rikvip
- B52
- 789CLUB
- X8 CLUB
- Nhất Vip
Câu hỏi thường gặp
Nhà cái tặng tiền miễn phí là gì?
Tiền cược miễn phí hay còn gọi là freebet chính là ưu đãi lớn của các nhà cái dành cho người chơi nhằm mục đích thu hút thêm nhiều người chơi hơn, mở rộng thêm cộng đồng cá cược online vốn đã vô cùng nhộn nhịp. Được nhận tiền khuyến mãi miễn phí để trải nghiệm hàng ngàn trò chơi cá cược online hấp dẫn thì quả là tuyệt vời đúng không nào?
Tuy nhiên thì tất nhiên các nhà cái không thể phát tiền một cách bừa bãi được. Người chơi vẫn phải thỏa mãn một số điều kiện riêng của từng nhà cái để nhận freebet. Đây không phải là keo kiệt vì khi tặng KM, rất có thể sẽ có nhiều người xấu vào trục lợi bằng cách tạo nhiều tài khoản ảo để nhận được nhiều ưu đãi, gây thất thoát. Vì thế để nhận được tiền cá cược miễn phí thì anh em chú ý phải thỏa mãn một số điều kiện rồi mới nhận tiền được.
Các khuyến mãi của các nhà cái mới nhất gồm:
- Game slot tặng tiền cược miễn phí, vòng quay miễn phí
- Casino tặng tiền cược miễn phí cho thành viên mới 2024
- Cá độ bóng đá, bảo hiểm thua cược với vé cược đầu
- Bắn cá đổi thưởng
Nhà cái tặng tiền không cần nạp 2024 có thật không?
Có nhiều tin đồn của một số người chơi là nhà cái lừa đảo, tung tin tặng tiền cược sau đó không thấy gì, chỉ tung tin để lừa người chơi truy cập. Nhưng sự thật hoàn toàn không phải vậy. Như tôi cũng đã nói ở trên, nhà cái sẽ tặng tiền trải nghiệm game miễn phí tuy nhiên phải thỏa mãn điều kiện riêng mới có thể nhận được thưởng để tránh tình trạng trục lợi lập nhiều tài khoản gây thất thoát hoặc bị lạm dụng.
Anh em yên tâm cứ làm theo đúng những hướng dẫn và yêu cầu của nhà cái chắc chắn sẽ nhận được KM nhé!
Đăng ký nhận code trải nghiệm game 2024 như thế nào?
Đây có lẽ là câu hỏi mà nhiều anh em thắc mắc nhất. Nhất là sau khi những phần trên tôi cứ nhắc đến những điều kiện và yêu cầu của nhà cái. Những thắc mắc đó của anh em sẽ được giải đáp ngay bây giờ.
Hiện nay, theo những thông tin mà tôi tìm hiểu được thì tùy từng nhà cái mà mức tiền cược và điều kiện sẽ có một chút khác nhau. Tôi sẽ chia sẻ anh em những cách đăng ký, điều kiện chung nhất của các nhà cái để có thể nhận quà nhé.
Cách để nhận code game là đăng ký trên website chính thức của nhà cái. Đăng ký thành công chưa đủ, anh em cần phải đăng ký tài khoản với đầy đủ thông tin thì mới được công nhận việc đăng ký của mình và như thế mới có thể nhận được. Cụ thể cần phải xác nhận một số thông tin sau:
Thông tin |
Hình thức |
Trạng thái |
✅ Thông tin hồ sơ cá nhân |
⭐ Bổ sung đầy đủ thông tin và chính xác |
Thành công |
✅ Thông tin chi tiết ngân hàng |
⭐ Điền đầy đủ và chính xác |
Thành công |
✅ Số điện thoại liên hệ đăng ký |
⭐ Xác nhận qua mã nhà cái gửi |
Thành công |
✅ Địa chỉ email đăng ký tài khoản |
⭐ Xác nhận qua mã nhà cái gửi |
Thành công |
Lưu ý về các nhà cái uy tín khuyến mãi thành viên mới 2024
Cũng có thêm một số lưu ý anh em cần chú ý để có thể nhận tiền cược một cách dễ dàng, không gặp nhiều rắc rối. Cụ thể những lưu ý của tôi dành cho anh em như sau:
- Tùy vào nhà cái có thể sẽ yêu cầu anh em phải nạp và rút một số tiền nhất định mới được nhận.
- Những thông tin được xác nhận ở trong bảng trên anh em cần điền đầy đủ, cẩn thận và chính xác vì nếu sai sẽ không được nhận tiền.
- Mỗi thành viên sẽ chỉ được đăng ký duy nhất 1 tài khoản và chỉ được nhận tiền đúng một lần.
- Tiền thưởng sẽ tự động chuyển vào tài khoản chơi của thành viên khi đáp ứng đủ yêu cầu.
- Tùy nhà cái mà anh em cần phải tham gia một số trận cược nhất định mới được rút tiền.
- Anh em cũng nên để ý những quy định chung về khuyến mãi nữa nhé.
Kết luận
Qua bài viết này mong rằng anh em đã có đầy đủ thông tin về tặng tiền cược miễn phí cùng như danh sách top 15 nhà cái tặng tiền miễn phí 2024. Mong rằng với khuyến mãi từ các nhà cái như thế này, anh em sẽ ngày càng hào hứng trải nghiệm hơn dịch vụ cá cược trực tuyến tiện lợi này.
bonesuk.com – trang chia sẻ code nhà cái uy tín – khuyến mãi freebet #1 VN
BÀI VIẾT MỚI NHẤT – BONESUK.COM
ĐÁNH GIÁ NHÀ CÁI
KINH NGHIỆM CÁ CƯỢC